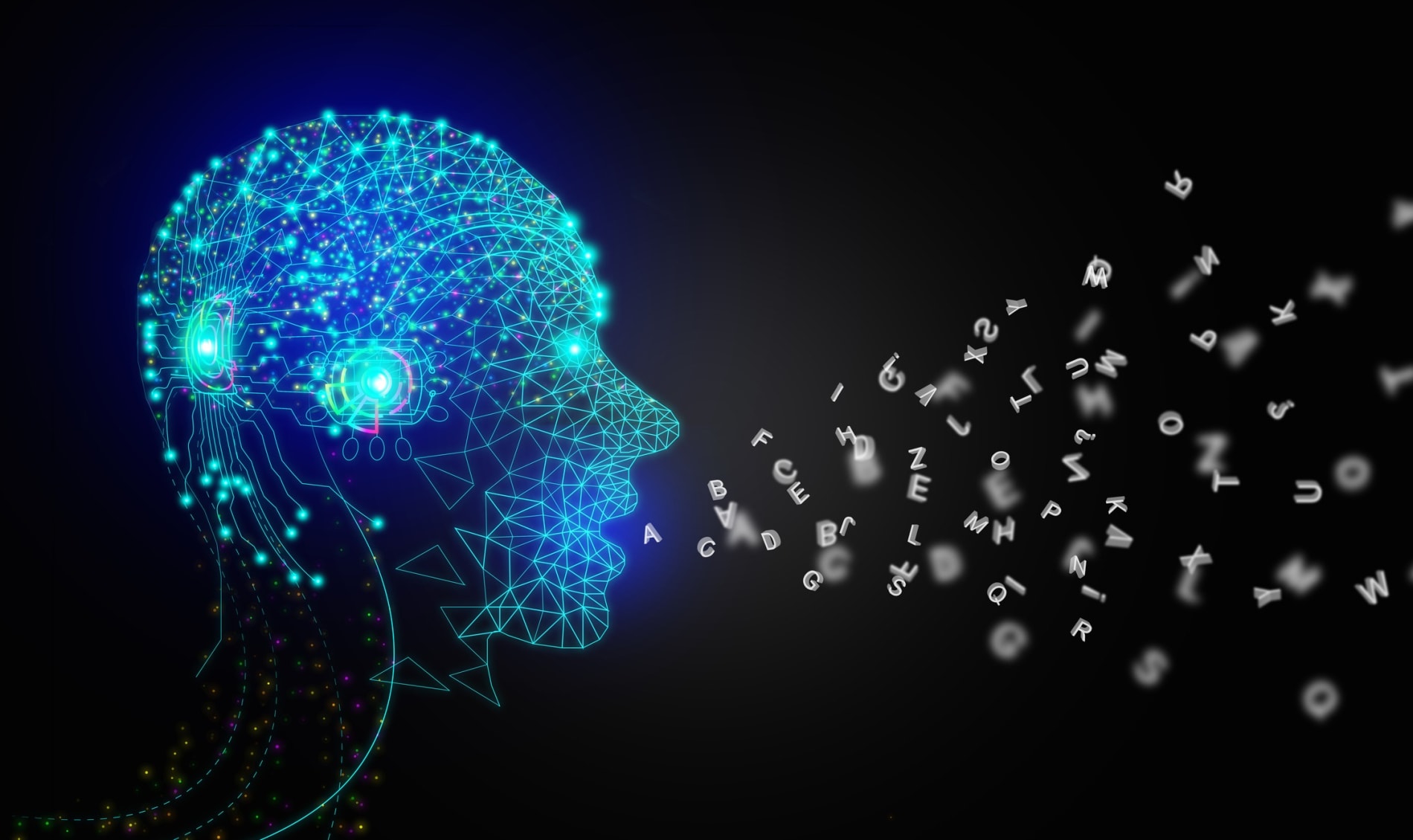
Within the quickly changing field of artificial intelligence, generative AI has become a revolutionary force that is changing our perception of and interactions with technology. This type of AI has revolutionized a number of industries, including data analysis, literature, and the arts.
It is renowned for its capacity to produce fresh, unique material, including writing and images. But when we marvel at what generative AI is capable of, an important issue comes to mind -can generative AI and data quality co-exist?
A key component of successful AI systems is data quality, which includes features like relevance, correctness, completeness, and dependability. It serves as the foundation for the construction and training of all AI models, including generative ones.
This article delves into the intricate relationship between generative AI and data quality. It investigates if there is an inherent conflict between these two essential elements of the contemporary data landscape or whether they may coexist peacefully.
What Is Generative AI?
Generative artificial intelligence can learn from existing artifacts in order to produce new artifacts that are realistic and realistic at scale. These artifacts mimic the features of the training data without repeating it. This technology is capable of producing a wide range of original material, including but not limited to photographs, videos, music, voice, text, software code, and product ideas.
Generative artificial intelligence makes use of a variety of methods that are constantly being improved upon. First and foremost are artificial intelligence foundation models, which are trained on an extensive collection of unlabeled data and may be used for a variety of tasks once additional fine-tuning has been performed.
These trained models are, in essence, prediction algorithms; nevertheless, the creation of these models requires a great deal of complex mathematics and a tremendous amount of processing power.
Currently, the most prevalent use of generative artificial intelligence is the creation of content in response to requests made in plain language. This type of AI does not require any prior knowledge of or entry into code. However, there are various corporate use cases, which include advancements in medication and chip design as well as material science development.
What Is Data Quality?
In order to guarantee that data is suitable for meeting the unique requirements of an organization in a given situation, quality management techniques are applied to data through the formulation and execution of various activities.
This process is known as data quality. High-quality data is defined as information that is judged appropriate for its intended use. Duplicate data, incomplete data, inconsistent data, erroneous data, poorly specified data, poorly structured data, and inadequate data security are a few examples of problems with data quality.
Data quality analysts carry out the evaluation and interpretation of each data quality measure, combine the scores to determine the overall quality of the data, and provide businesses with a percentage that indicates how accurate their data is.
A low data quality scorecard is indicative of low-quality data, which has little value, is deceptive, and can result in bad decisions that could be detrimental to the company.
Data governance is the act of creating and implementing a specified, mutually agreed-upon set of guidelines and standards that control all data inside an organization. Data quality criteria are a crucial part of this process.
Inconsistencies and errors that might impair the accuracy of data analytics and regulatory compliance should be eliminated, and data from diverse data sources should be harmonized. Effective data governance should also establish and enforce data usage standards.
Dimensions Of Data Quality
Numerous criteria are used to evaluate data quality, and these criteria might vary depending on the information's source. Data quality measures are categorized using these dimensions;
Completeness
This is a representation of the total amount of whole or valuable data. If the data is not representative of a regular data sample, a large percentage of missing values might result in a skewed or misleading conclusion.
Uniqueness
This explains how much duplicate data there is in a dataset. For instance, you should anticipate that every client has a distinct customer ID while evaluating customer data.
Authenticity
The degree to which data adheres to the format necessary for any business requirements is measured by this dimension. Metadata, including appropriate data types, ranges, patterns, and more, are typically included in formatting.
Timeliness
This dimension refers to the preparedness of the data within the anticipated time range. For instance, order numbers must be created in real time as customers expect to get them as soon as they complete a transaction.
Precision
Since there may be several sources reporting on the same metric, it's vital to select a primary data source; additional sources can be utilized to check the accuracy of the primary one.
This dimension pertains to the correctness of the data values based on the agreed-upon "source of truth." To increase trust in the accuracy of the data, technologies can, for instance, verify that all data sources are going in the same direction.
Continuity
This dimension evaluates two distinct datasets' worth of data records. It is possible to find several sources reporting on the same measure, as was previously indicated. Employing many sources to verify recurring patterns and conduct in data enables companies to have confidence in any practical findings derived from their inquiries.
This reasoning may also be used to analyze data relationships. For instance, a department's workforce size should be, at most, the company's overall workforce size.
Fitness For Purpose
Last but not least, fitness of purpose guarantees that the data asset satisfies a business requirement. It might be challenging to assess this dimension, especially when working with recently developed datasets.
With the use of these metrics, teams may examine the informativeness and utility of data for a particular purpose within their businesses.
How Does Generative AI Rely On High-Quality Data?
A game-changer in the field of artificial intelligence, generative AI can produce a wide range of fresh, creative outputs, from text and images to music and code. Its transformative potential stems from its fundamental dependence on high-quality data.
The crucial significance of high-quality data for the efficiency and proper operation of generative AI systems is explored in this portion of the paper. Any successful AI system must have high-quality data, but generative models like DALL-E and GPT (Generative Pre-trained Transformer) really need it.
Through the identification of patterns, correlations, and structures in the training data, these models acquire the ability to produce new material. The caliber of this training data directly affects the model's capacity to generate impartial, accurate, and relevant outputs.
Data's Function In Generative AI Training
Large datasets are commonly used to train generative AI models. These datasets need to be high in quality and sizable as well. In this context, accurate, diversified, complete, and representative data of the real-world scenarios the model would face are considered high-quality data.
For example, to effectively learn and emulate human writing, a generative AI model taught to produce articles needs data from a wide range of themes written in diverse styles and tones.
Data Quality's Effect On AI Results
The caliber of the data used to train AI directly affects the output that it produces. The AI's outputs are likely to reinforce any biases present in the training set.
Similarly, the AI will find it challenging to produce logical and trustworthy results if the data is full of mistakes or contradictions. This is especially true for language models, where low-quality data might produce text that needs to be factually accurate or incomprehensible.
Difficulties In Maintaining Data Quality
The vast amount of data needed to train these models is one of the major obstacles to maintaining data quality for generative AI. It is a difficult undertaking to make sure that such large volumes of data adhere to quality requirements.
Furthermore, because generative AI models are frequently applied in dynamic settings, the training data must be updated and verified on a regular basis to ensure its accuracy and relevance.
Data Integrity And Ethical Issues
There are ethical ramifications for data quality as well. For example, a generative AI model may unintentionally reinforce gender or racial prejudices in its outputs if it is trained on data containing such biases.
Therefore, maintaining data quality is not only technically necessary but also morally required to avoid perpetuating stereotypes and societal biases. The importance of having high-quality data for generative AI cannot be emphasized.
The quality of data used to train these models has a significant impact on the efficacy, dependability, and moral purity of the outputs produced by generative AI. Making investments in and giving priority to high-quality data will be crucial to maximizing the potential of generative AI technologies as the field of artificial intelligence continues to progress.
Use Cases For Generative AI In Data Quality
Data Augmentation
By creating additional samples that are variants of preexisting data, a technique known as "data augmentation" may be used to enhance the size of a dataset artificially. This strategy enhances the resilience of machine learning models and helps to diversify the data. Additional data points that are compatible with the original distribution can be produced by using generative AI.
Generative AI, for instance, may be used to generate fresh photos with varied lighting, orientations, or backdrop settings for image recognition tasks, which improves the model's generalization capacity.
We may use the Image Classification for Medical Diagnoses as a real-time example. Consider a medical imaging application that uses X-rays to determine diseases like fractures of the bones.
Generative AI may enhance the dataset through the generation of X-ray picture variants with varying lighting conditions, angles, and slight distortions. An image classification algorithm that is more resilient to a variety of picture properties can detect fractures with more accuracy thanks to this enhanced dataset.
Anomaly Detection
By discovering deviations from the typical patterns and structures present in a dataset, generative AI may help in anomaly identification. A generative AI model may discover the underlying distribution of average data points by training on clean data.
The model may then identify examples that significantly depart from the learned distribution as possible anomalies when it is exposed to new data. This method works exceptionally well for quality assurance, network security, and fraud detection. Let's consider a system for detecting credit card fraud.
A significant risk in the banking sector is credit card theft. In real-time data streams, generative AI may detect departures from the patterns learned from genuine transactions. The system may identify a transaction as fraudulent if it deviates considerably from the learned distribution of typical transactions. This allows for quick action to stop any unlawful charges.
Synthetic Data Generation
Generative AI may be used to create synthetic data that resembles the features of the original dataset in scenarios where accurate data is sensitive or complex to get. After creation, this fake data may be shared, tested, and used to construct models without revealing accurate, private information.
This strategy is beneficial in sectors where privacy is a top priority, such as healthcare, banking, and other industries. Strict privacy protections are necessary for sensitive data, such as financial transactions or medical records. By creating synthetic data that imitates the original while protecting privacy, generative AI steps in to save the day.
For example, without jeopardizing patient anonymity, medical researchers can do tests using artificial patient data. The utilization of synthetic data facilitates advancements in machine learning by enabling developers to refine models without jeopardizing confidential data.
Natural Language Processing (NLP) Data Quality
The problems with data quality connected to NLP can be improved with the use of generative AI. For instance, it can help provide text that has been paraphrased in order to produce a variety of training datasets for language models.
Furthermore, generative AI may assist with text completion tasks by recommending words or phrases that are contextually appropriate to improve the readability and coherence of text data.
Chatbots, virtual assistants, and language translation systems are powered by natural language processing or NLP. By producing paraphrased text or coherently finishing phrases, generative AI improves natural language processing (NLP).
Imagine a chatbot for customer service. Customer interactions become more fluid and efficient because of generative AI's guarantee of providing a variety of contextually relevant and diverse replies.
Data Cleansing
Finding and fixing mistakes, inconsistencies, and inaccuracies in a dataset is known as data cleaning. In order to help with this process, generative AI may provide logical substitute values for inaccurate or missing data points.
For example, generative AI with text data can provide appropriate substitutions for misspelled words or missing values by analyzing the surrounding textual context. Data cleaning is the most common use of generative AI in the field of data quality.
Generative AI may be utilized for the following data quality issue resolution in a Master Data Management System (for instance, a Customer Master Application).
Strategies For Co-Existence Of Generative AI And Data Quality
Developing coexistence techniques between generative AI and data quality is essential in the dynamic interplay between the two. Establishing strong data governance structures is a crucial tactic.
Clear guidelines for data collection, validation, storage, and use should be included in these frameworks to guarantee that the high-quality, ethically generated data that powers AI systems is used. Constant data set monitoring and upgrading is another essential strategy.
The data that generative AI uses has to be updated to reflect new developments and avoid obsolescence. Regular audits are part of this process to check for bias, relevance, and correctness.
Integrating human monitoring is also crucial. Even while AI is capable of processing and analyzing data at previously unheard-of levels, human judgment is still crucial for making complicated or delicate decisions.
By working together, it will be possible to detect and address biases, guarantee that ethical standards are upheld, and preserve the general caliber of the information produced by AI. Furthermore, encouraging explainability and openness in AI operations strengthens relationships and makes it easier to comprehend and manage AI procedures.
Clear documentation of AI models, their training sets, and decision-making procedures can help achieve this. Last but not least, providing teams in charge of AI systems with ongoing education and training guarantees that they will be competent in managing changing data landscapes and technology.
Frequently Asked Question
Can Generative AI Improve The Quality Of Its Training Data Over Time?
Yes, generative AI can be designed to enhance data quality over time through continuous learning and updating, but this requires initial high-quality data and ongoing monitoring.
Are There Specific Challenges In Maintaining Data Quality For Generative AI?
Yes, challenges include managing the vast volume of data needed, ensuring diversity and representativeness, and continuously updating the data to maintain its relevance.
How Does Poor Data Quality Affect The Outputs Of Generative AI?
Data quality can lead to accurate, biased, or irrelevant outputs from generative AI, undermining its reliability and effectiveness.
Conclusion
The question "Can generative AI and data quality co-exist?" finds its answer in the affirmative, albeit with concerted efforts and strategic approaches. The symbiotic relationship between generative AI and data quality is not only possible but essential for the advancement of reliable and ethical AI technologies.
By prioritizing high-quality data, implementing robust governance frameworks, and ensuring continuous human oversight, we can harness the full potential of generative AI.
This co-existence is pivotal in shaping a future where AI not only innovates and automates but does so with accuracy, fairness, and integrity, reflecting the best of technological advancement and data stewardship.